The role of Artificial Intelligence in revolutionizing renewable energy. Photo Courtesy – Pexels
Introduction: In recent years, renewable energy has emerged as a vital solution to combat climate change and transition to a sustainable future. Simultaneously, the development of artificial intelligence (AI) has revolutionized various industries, from healthcare to transportation. Now, AI is making significant strides in the renewable energy sector, transforming the way we generate, manage, and utilize clean energy. By harnessing the power of AI, we can accelerate the adoption of energy sources, optimize energy systems, and create a greener and more efficient world. In this article, we will explore the impact of AI on renewable energy and the key areas where AI is being applied to revolutionize the sector.
Enhancing Renewable Energy Generation:
AI plays a crucial role in optimizing renewable energy generation. Machine learning algorithms can analyze vast amounts of data, such as weather patterns, historical energy production, and grid demand, to improve the efficiency and output of renewable energy sources. For instance, AI can analyze meteorological data to predict wind patterns and optimize the operation of wind farms, maximizing energy production. Similarly, solar power plants can benefit from AI algorithms that predict cloud cover and adjust solar panel positioning for optimal sunlight absorption. By leveraging AI, renewable energy generation can become more reliable, predictable, and economically viable.
Smart Grids and Energy Management:
AI-enabled smart grids are transforming the way we manage and distribute energy. Traditional power grids face challenges such as fluctuating demand, intermittent renewable energy sources, and aging infrastructure. AI algorithms can analyze real-time data from smart meters, sensors, and energy consumers to optimize energy distribution, predict demand, and balance supply and demand in real time. These systems help reduce energy wastage, prevent blackouts, and enable a more resilient and flexible energy infrastructure. Additionally, AI algorithms can optimize charging schedules for electric vehicles, reducing peak demand and integrating them into the grid seamlessly.
Read: The unexpected presence of earthworms at the North Pole may cause changes in ecosystems
Energy Efficiency and Demand-Side Management:
AI is instrumental in promoting energy efficiency and demand-side management. Machine learning algorithms can analyze energy consumption patterns in buildings, industries, and homes to identify energy-saving opportunities. By using AI-powered systems, buildings can optimize heating, cooling, and lighting, reducing energy waste while maintaining comfort levels. AI algorithms can also provide real-time energy feedback, enabling consumers to make informed choices about their energy usage. Furthermore, demand-side management techniques, combined with AI, can dynamically adjust energy consumption to match renewable energy availability, reducing the reliance on fossil fuel-based power plants during peak demand periods.
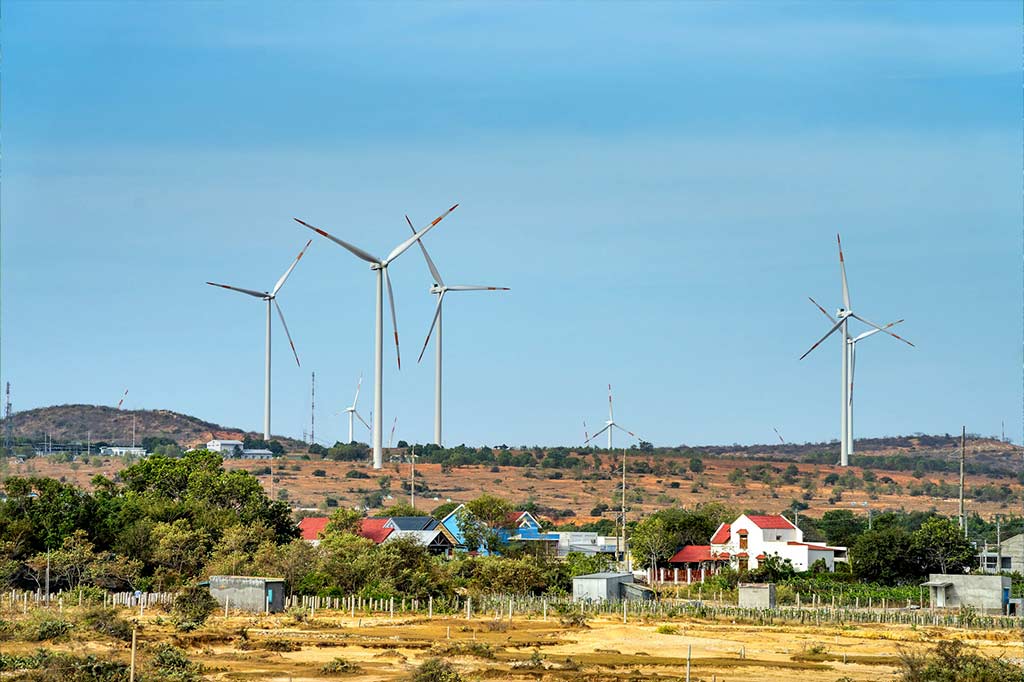
Various aspects and process of renewable energy incorporating AI. Photo Courtesy – http://www.ibnlive.com.
Predictive Maintenance and Asset Optimization:
Maintaining and optimizing renewable energy infrastructure is vital for long-term sustainability. AI can assist in predictive maintenance by analyzing real-time sensor data, historical maintenance records, and machine-learning models. This approach helps detect potential failures or performance issues in renewable energy assets such as wind turbines, solar panels, and battery storage systems. By predicting maintenance needs in advance, AI can optimize repair schedules, reduce downtime, and maximize the lifespan of renewable energy infrastructure.
Energy Forecasting and Market Analysis:
Accurate energy forecasting and market analysis are crucial for planning and integrating renewable energy into the grid effectively. AI algorithms can analyze historical energy data, weather patterns, and market conditions to predict future energy demand and optimize energy production schedules. Such forecasts can guide decision-making for policymakers, grid operators, and renewable energy investors. AI-powered market analysis can also identify opportunities for renewable energy investment, enabling the growth of a more sustainable energy economy.
AI implementation in renewable energy can be both costly and cost-effective, depending on various factors. Here’s a breakdown of the cost considerations:
Implementation Costs: Introducing AI systems in the renewable energy sector involves upfront costs such as acquiring AI technology, hardware, software, and the necessary infrastructure to support it. Developing or acquiring AI algorithms and models tailored to specific energy applications may also require investment. Additionally, training personnel or hiring AI experts to manage and operate these systems adds to the initial costs.
Data Collection and Processing: AI systems rely heavily on data for training and operation. Collecting and processing large volumes of real-time data from energy sources, weather patterns, energy consumption, and grid conditions can incur expenses. This includes installing sensors, smart meters, and communication networks to gather data. Moreover, the computational power required for processing and analyzing this data may necessitate additional investments.
Read: Pancreatic cancer caused by PPP1R1B protein, Dr Anindya Bagchi says
Operational and Maintenance Costs: After implementation, there are ongoing operational and maintenance costs associated with AI systems. These include regular updates and improvements to algorithms, managing data storage and processing infrastructure, and training personnel to ensure efficient operation. The complexity of AI systems may require skilled technicians and engineers to maintain and troubleshoot them, adding to the operational costs.
Despite these costs, AI can ultimately prove cost-effective in renewable energy applications due to its potential benefits:
Efficiency Improvements: AI can optimize renewable energy systems by enhancing generation, reducing wastage, and improving overall efficiency. By maximizing energy production, minimizing downtime, and enhancing energy distribution, AI can help reduce costs associated with suboptimal operations and improve the financial viability of renewable energy projects.
Predictive Maintenance: AI’s ability to predict maintenance needs and potential failures in energy infrastructure enables proactive maintenance, reducing downtime and costly emergency repairs. By optimizing maintenance schedules, AI helps prevent unexpected failures and ensures the longevity and reliability of energy assets, ultimately lowering maintenance costs.
Demand-Side Management: AI-powered demand-side management allows better control and optimization of energy consumption, reducing peak demand and mitigating the need for expensive additional power generation capacity. By dynamically adjusting energy usage patterns, AI can help optimize energy costs and grid stability.
Market Analysis and Forecasting: Accurate energy forecasting and market analysis facilitated by AI can guide decision-making in renewable energy investments. By identifying the most cost-effective projects, predicting market trends, and optimizing energy production schedules, AI can reduce financial risks and improve returns on investment.
It is important to note that the cost-effectiveness of AI in renewable energy also depends on the scale of implementation, the specific application, and the local context. In some cases, the initial costs of implementing AI systems may be outweighed by long-term benefits, such as increased energy production, reduced operational expenses, and enhanced grid efficiency. Continuous advancements in AI technology and economies of scale may also contribute to cost reductions over time.
Overall, while there are initial costs associated with implementing AI in this energy process, the potential long-term benefits and cost savings make it a promising investment for a sustainable and efficient energy future.
Conclusion: The synergy between artificial intelligence and renewable energy holds immense promise for a greener and more sustainable future. By leveraging AI algorithms, we can optimize this energy generation, improve energy management and efficiency, enhance grid stability, and transform the way we produce and consume energy. As AI continues to evolve, it is crucial to prioritize transparency, ethics, and accountability to ensure responsible and equitable deployment of these technologies. With continued advancements, AI-driven solutions have the potential to accelerate the global transition to renewable energy, mitigating climate change, and creating a cleaner and more resilient energy ecosystem.